Paper analysis “Prometheus - Inducing Fine-grained Evaluation Capability in Language Models”
Table of Contents
The Why?

Evaluating RAG-based chatbot performance can be challenging, particularly when diverse customer datasets are at play. The central question is: How do we measure the quality of a conversation when the parameters of ‘good’ are so variable? In the previous article, I proposed a simple method which was based on generating synthetic questions from customer data, and then we were able to measure quality using RAGAs. This involved a two-fold process where GPT-4 not only generated responses but also served as a judge to evaluate their quality based on the context provided. While this method is quick to implement and sets a good baseline, it has many limitations.
Limitations
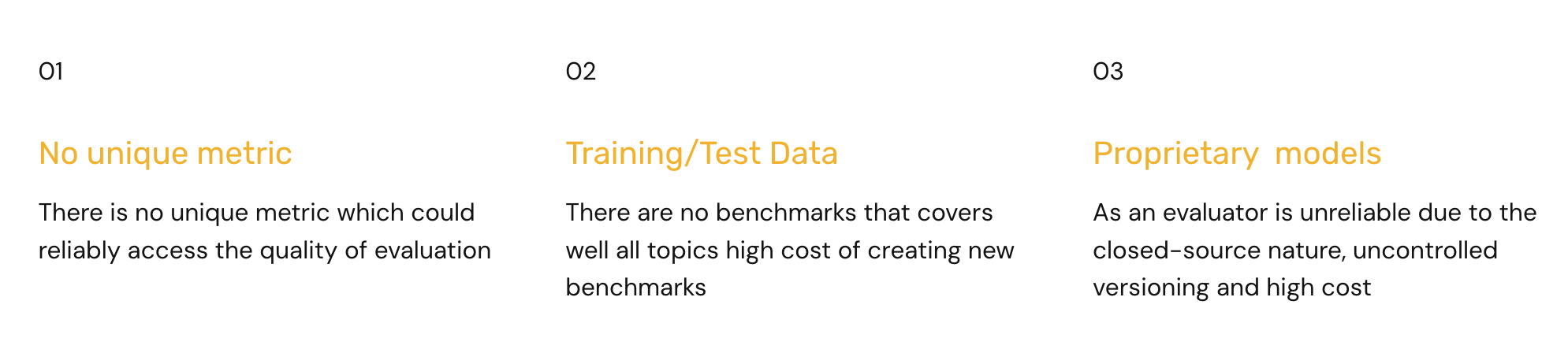
- Unreliability of existing metrics: Our chosen metric, RAGAS, proved to be inconsistent, leading to doubts about its effectiveness in providing a true measure of quality. RAGAS gives only a score in the range from 0 to 1, which is difficult to interpret and has built-in bias from LLM itself.
- Speed: The evaluation process was time-intensive. Multiple interactions with GPT-4 for each question and its subsequent judgment took 30-45s on average, making it slow to test new ideas frequently.
- Cost Considerations: Frequent use of GPT-4’s evaluative capacity came with substantial costs, particularly when the evaluations were for internal purposes rather than customer-driven.
To mitigate these issues, we have adopted parallel execution and threading to expedite the process. However, to manage costs, we have limited the frequency of evaluations to periods of significant change.
Is there a better approach?
There are many papers that tries to work on the limitations discussed above. Some of them are:
- Prometheus: Inducing Fine-grained Evaluation Capability in Language Models
- JudgeLM: Fine-tuned Large Language Models are Scalable Judges
- G-EVAL: NLG Evaluation using GPT-4 with Better Human Alignment
- Can Generalist Foundation Models Outcompete Special-Purpose Tuning? Case Study in Medicine
I got inspired by the work “Prometheus: Inducing Fine-grained Evaluation Capability in Language Models”. The cornerstone of this paper is its innovative approach to generating evaluation datasets based on rubric metric and finetuning local LLM on it. This enables the construction of a specialized Judge Language Model (LLM) that operates on open-source principles and is fine-tuned for nuanced assessment.
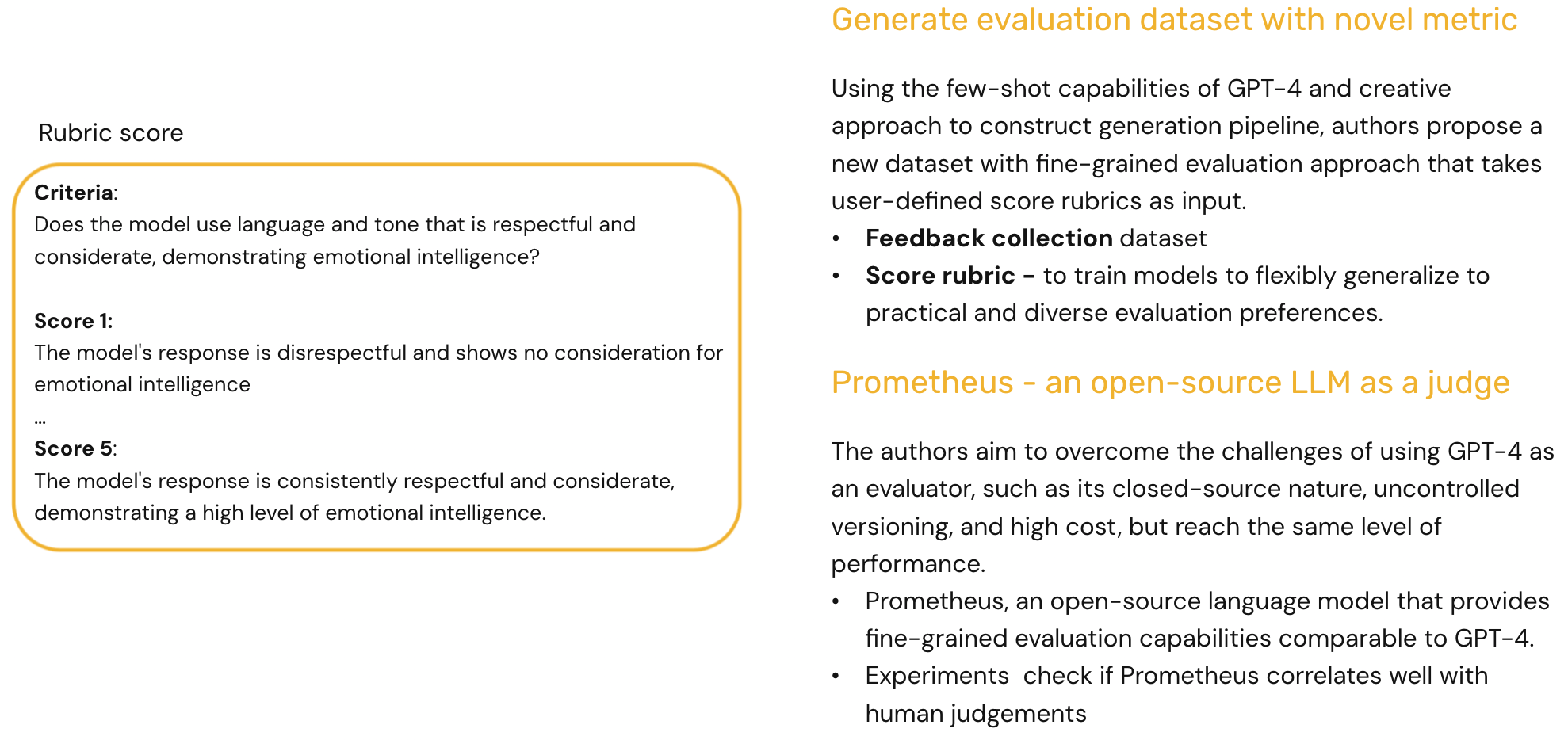
- GPT-4’s Breadth vs. Specialization: While GPT-4’s training encompasses a vast breadth of knowledge, our evaluation needs are often more focused. We’re interested in aspects like text length, tone of voice, and politeness of responses.
- Introducing the Rubric Score: The authors introduce a ‘rubric score’ to capture these fine-grained criteria. For instance, the rubric asks, “Does the model use language and tone that is respectful and considerate, demonstrating emotional intelligence?” Responses are then rated on a scale, with a score of 1 for disrespectful and emotionally unintelligent replies, and a score of 5 for consistent respectfulness and high emotional intelligence.
An open-source LLM as a judge
The authors assert that by utilizing this approach, performance can match that of GPT-4. This might sound ambitious, but it’s based on the principle that specialized models can outperform more generalist ones in specific tasks.
- Benefits of Specialization:
- Cost-Effectiveness: Moving away from GPT-4’s framework means reduced costs as the model can be hosted locally.
- Speed: Control over execution speed is gained, and scaling can be done by adding more machines.
- Consistency: The specialized model ensures consistent responses, provided no changes have been made to the system.
Data Structure
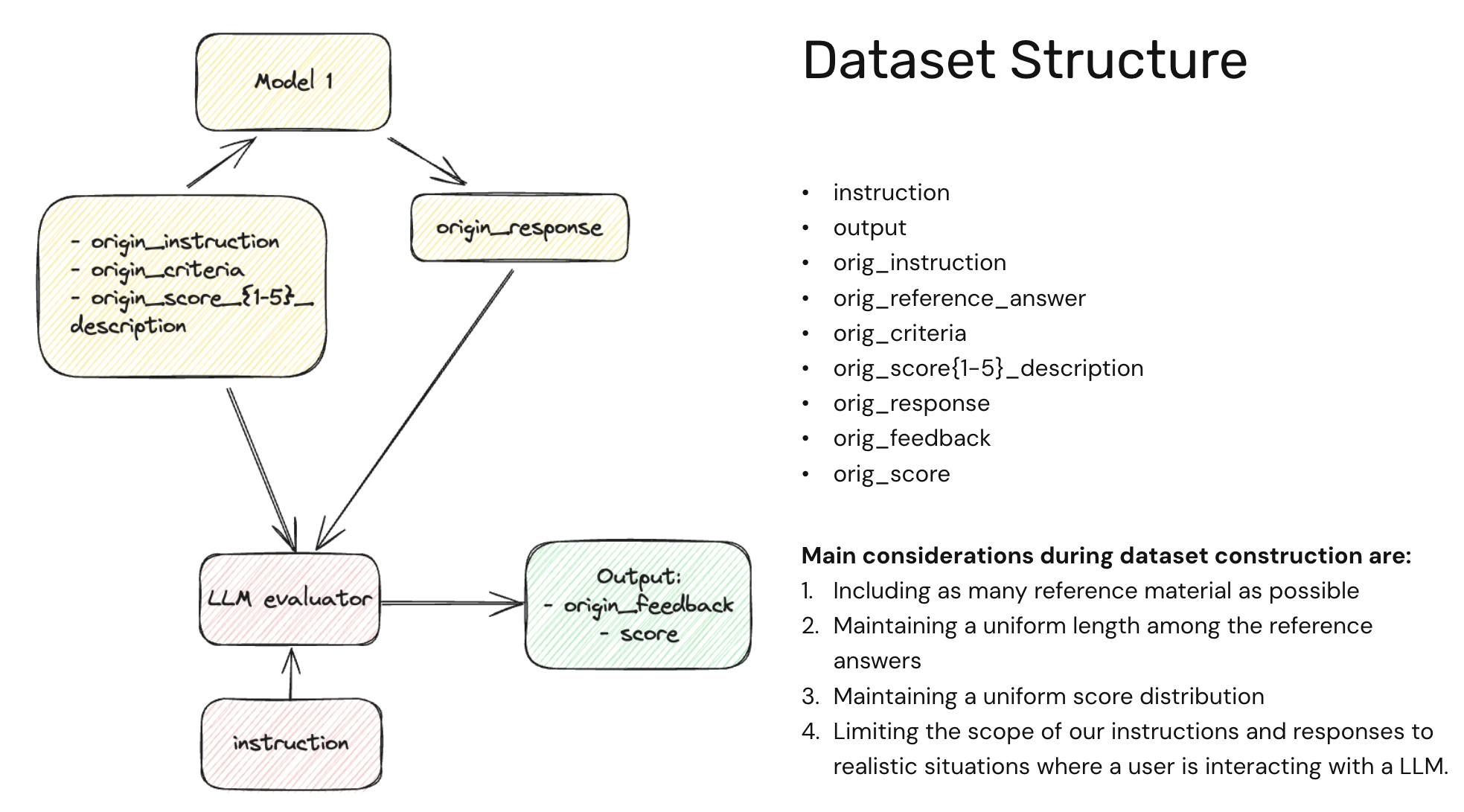
The Evaluation Model in Action
We start with Model 1, which could be our chatbot. The evaluation process is initiated with specific instructions that set the context for the chatbot’s task. These instructions are aligned with criteria and a scoring system ranging from 1 to 5, with descriptions for each score, for example reflecting the degree of emotional intelligence and respectfulness in the response.
Here’s a step-by-step breakdown:
- Instruction Input: An instruction is provided to the chatbot, which could be a request for information or a customer service inquiry.
- Criteria Definition: Accompanying the instruction is a set of criteria that outlines the expectations for the chatbot’s response.
- Original Response: The chatbot processes the instruction, user_input, scorining_criterias and responds accordingly.
- Evaluation by LLM Evaluator: A third-party evaluator, the JudgeLLM, then assesses the chatbot’s response against the criteria using a rubric score. The JudgeLLM provides feedback on the response and assigns a score based on the predefined criteria.
Main Considerations During Dataset Construction
To construct a robust dataset, we focus on:
- Comprehensive Reference Material: Incorporating a wide array of examples to cover various scenarios.
- Uniform Reference Length: Ensuring that the length of reference answers is consistent. This is important because LLMs frequently score long answers with higher score, even though the content is not good.
- Balanced Score Distribution: We would like to generate dataset with scores that have a meaningful descriptions: Score 1 is bad and progressively improving it until Score 5 which suppose to be a target answer.
- Scope Limitation: Focusing on realistic situations where a user interacts with a chatbot, ensuring that the instructions and responses are relevant and practical.
The Four-Step Process to Constructing a Robust Evaluation Dataset
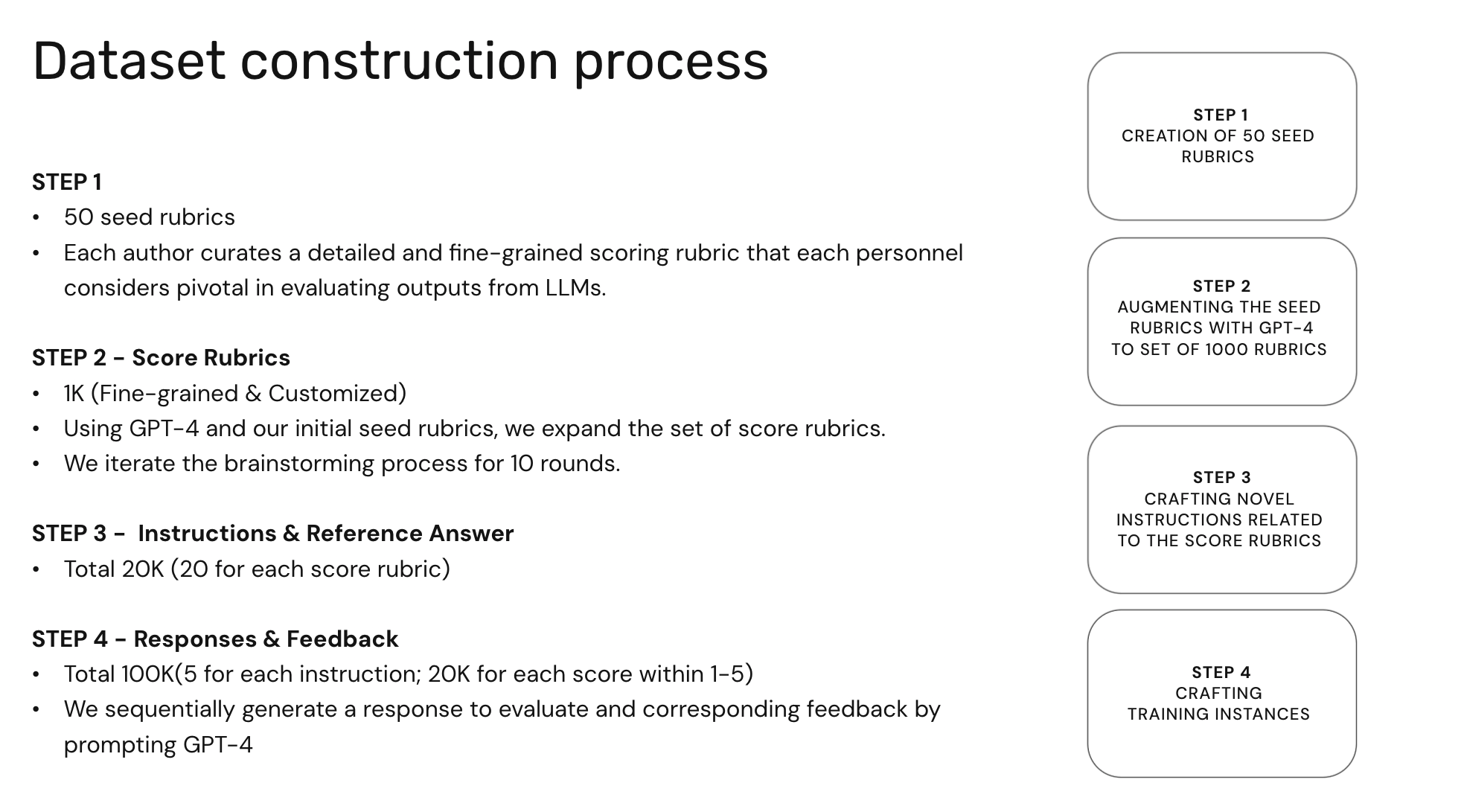
Creating an evaluation dataset from scratch can be a daunting task. However, the methodology introduced in the paper offers a structured and scalable approach. Let’s dive into the four-step process they’ve outlined.
Step 1: Seed Rubrics Creation
- Initially, 50 seed rubrics were handcrafted by the authors. These rubrics serve as the foundational examples that capture essential evaluation criteria for LLM outputs.
Step 2: Rubric Expansion
- To expand upon the initial seed rubrics, the authors utilized GPT-4. By inputting a random selection of four seed rubrics, GPT-4 was prompted to generate additional rubrics, thereby increasing the number to 1,000. This iterative process of brainstorming was repeated for 10 rounds to refine and diversify the rubrics.
Step 3: Generating Instructions and Reference Answers
- With the expanded set of rubrics, the next step was to generate instructions and reference answers. Each rubric was used as a prompt to create corresponding instructions and reference answers, resulting in a total of 20,000 instances (20 for each score rubric).
Step 4: Response and Feedback Generation
- The final step involved generating responses and feedback for each instruction and reference answer. This was done sequentially, with GPT-4 providing both the response and the feedback based on the previously generated rubrics and reference answers. The result was an extensive dataset of 100,000 instances (5 for each instruction, with 20K for each score within 1-5).
Fine-tuning an evaluator LM
The original code could be found here. The following prompt format (already processed in the ‘output’) was used to train the evaluator LM:
{orig_feedback} [RESULT] {orig_score}
Then during evaluation, we parsed the prediction after the phrase [RESULT].
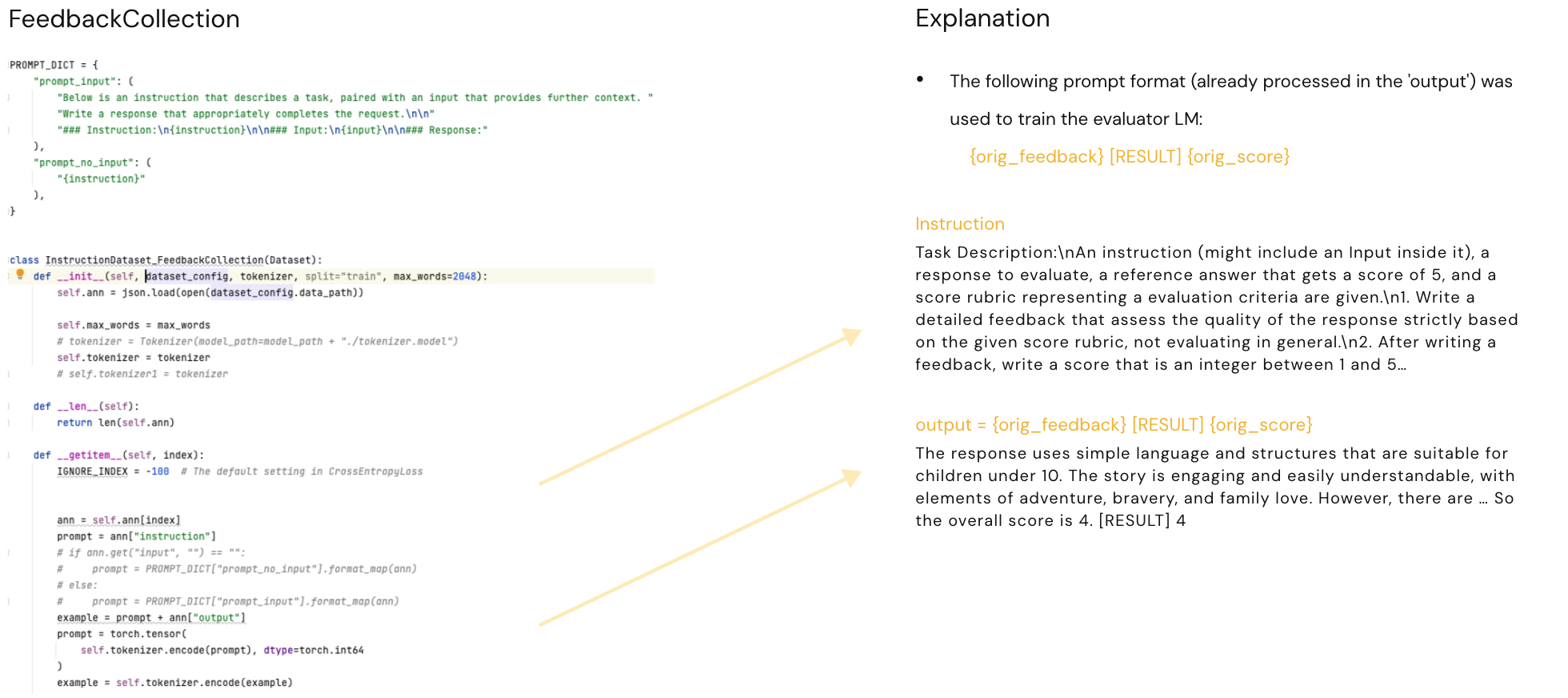
Finetuning
- 8xA100 (80GB) GPUs
- Full fine tuning
- Fully-Sharded Data Parallel (FSDP)
- They did not mask [instruction] part → Loss is only calculated on the feedback and score decision, not the [instruction]
- Training batch size which was set according to the model size: for 7B models we used 28 and for 13B models we used 20
- Number of epochs is set to 3
Evaluation Datasets
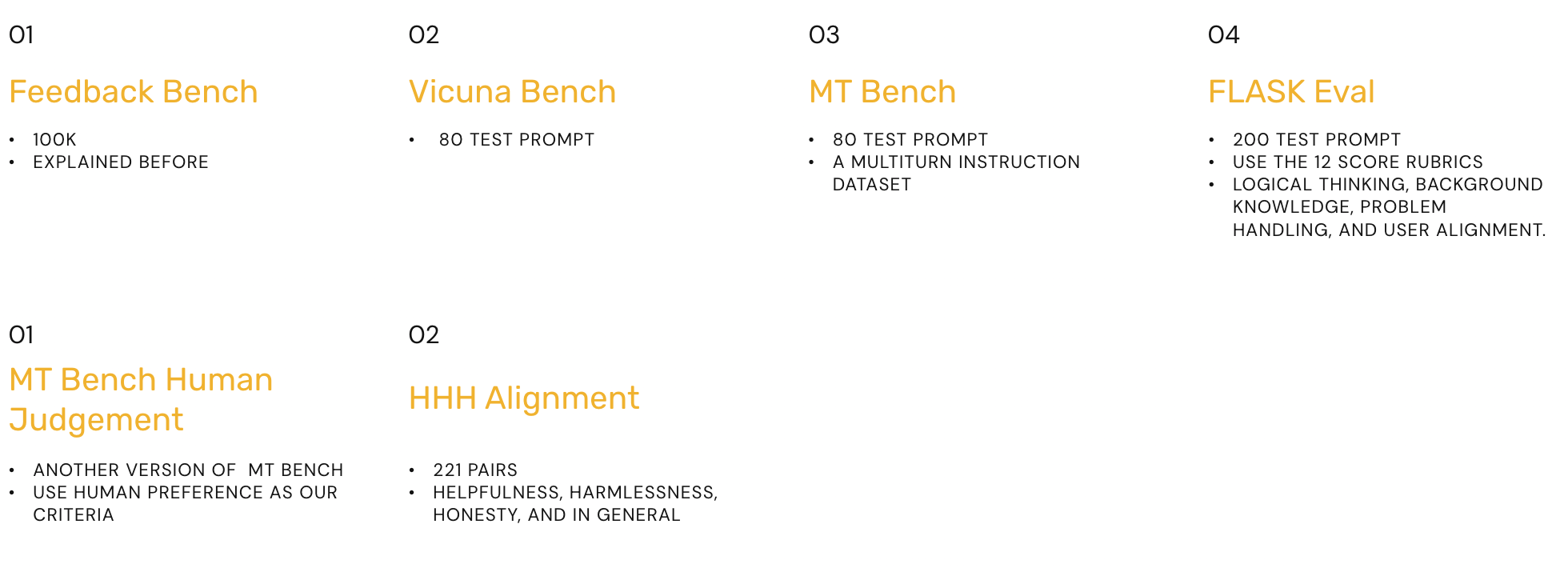
Results
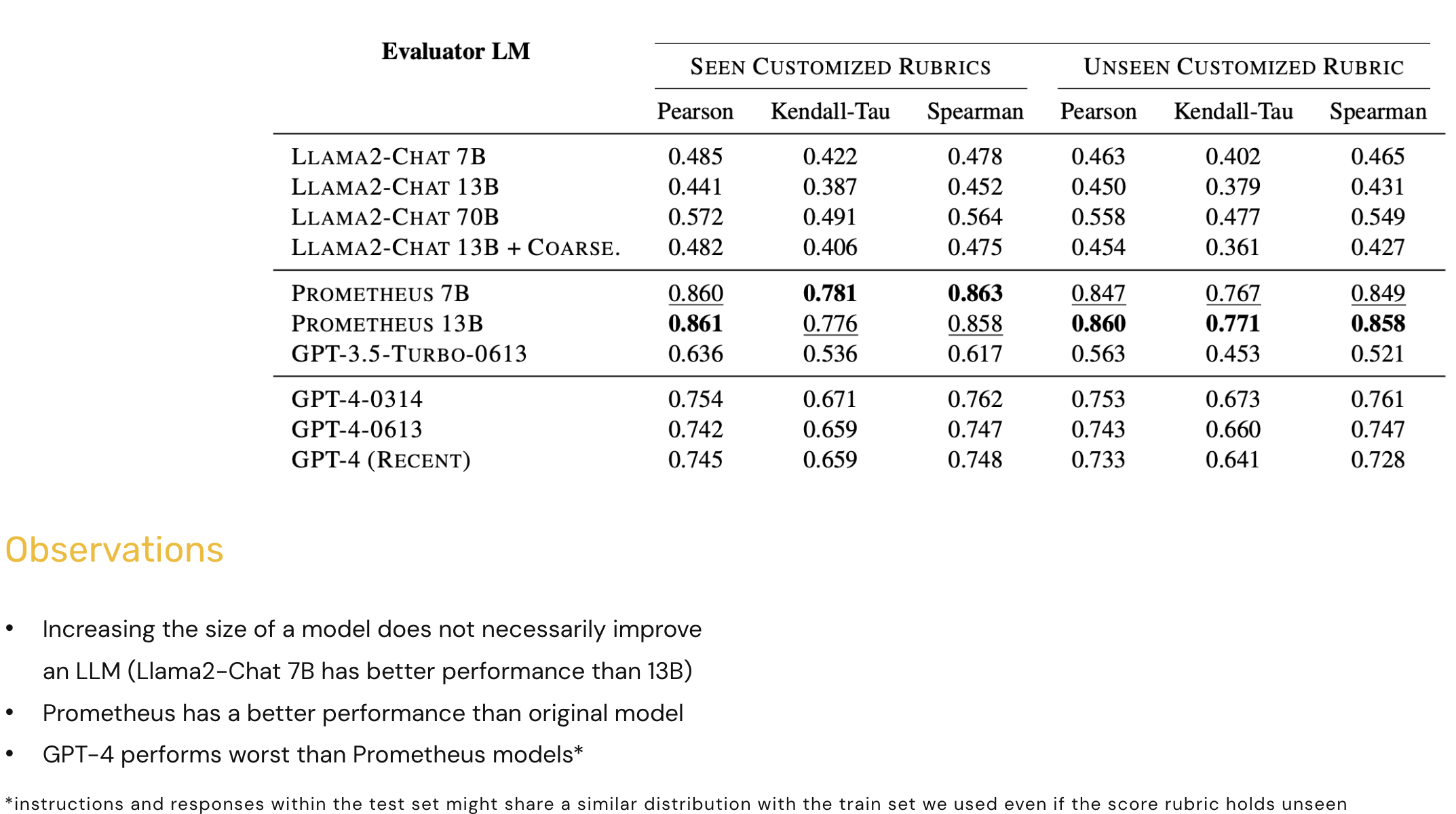
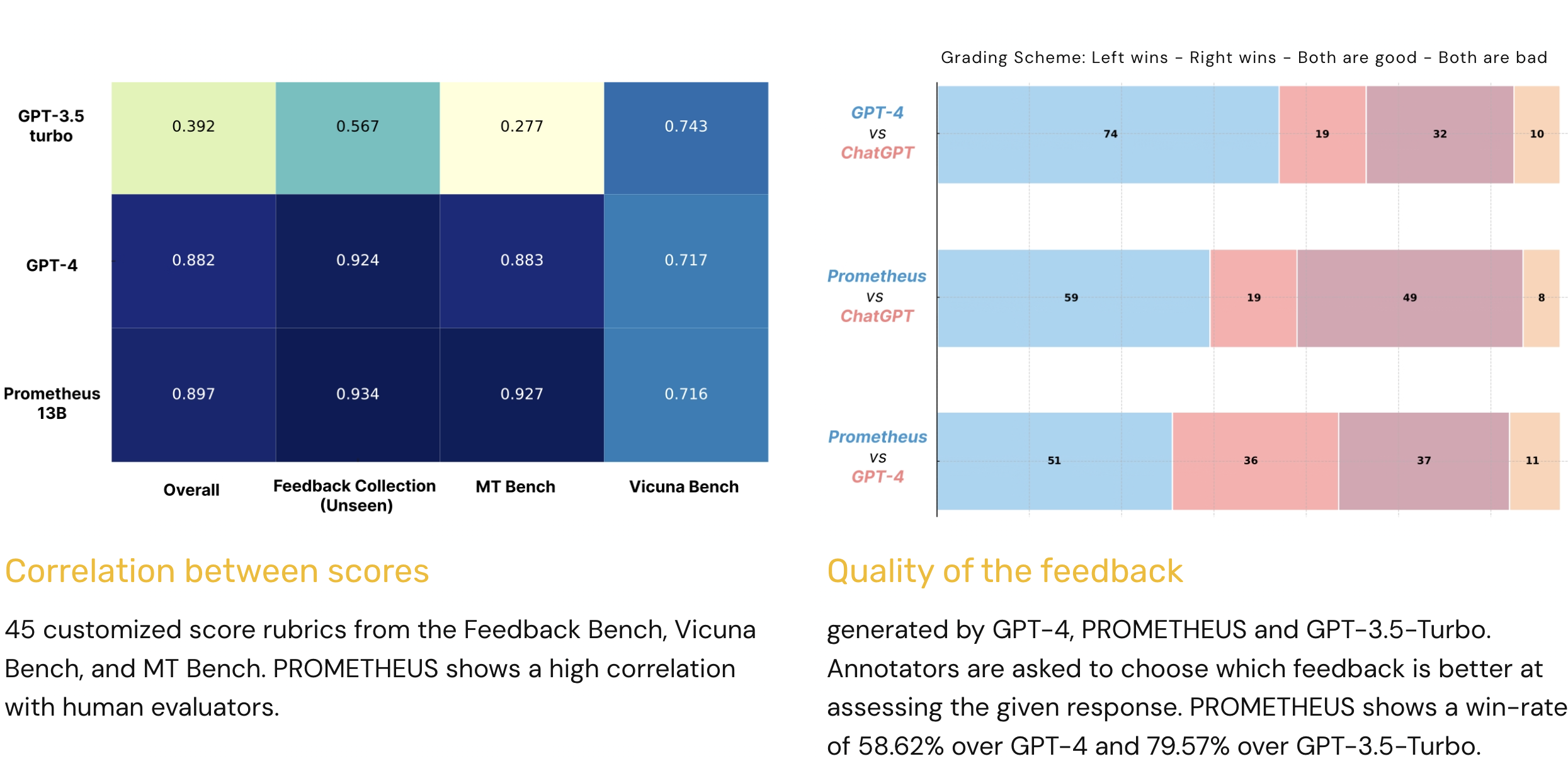
Summary
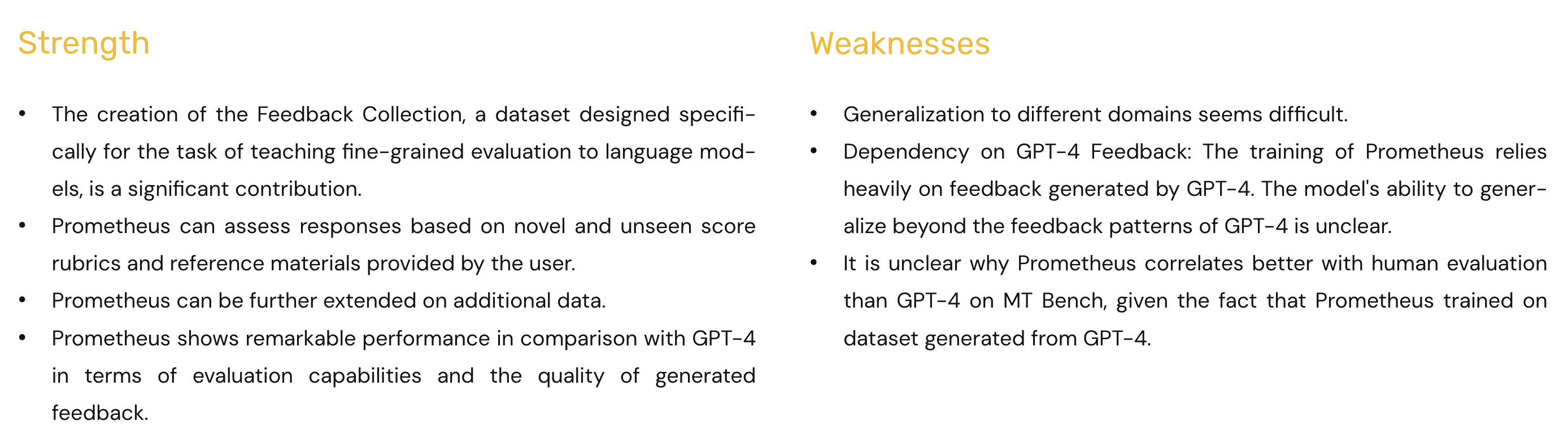
Code
In order to reproduce paper results, we can follow the original code. However, I prefer the implementations using Langchain, which can be found here and here. To perform finetuning, authors do it on their own clusters. I experimented with two platforms for fine-tuning. They were both user-friendly and reasonably priced: OpenPipe and TogetherAI.