How to build a dataset to evaluate a RAG?
Table of Contents
System Design
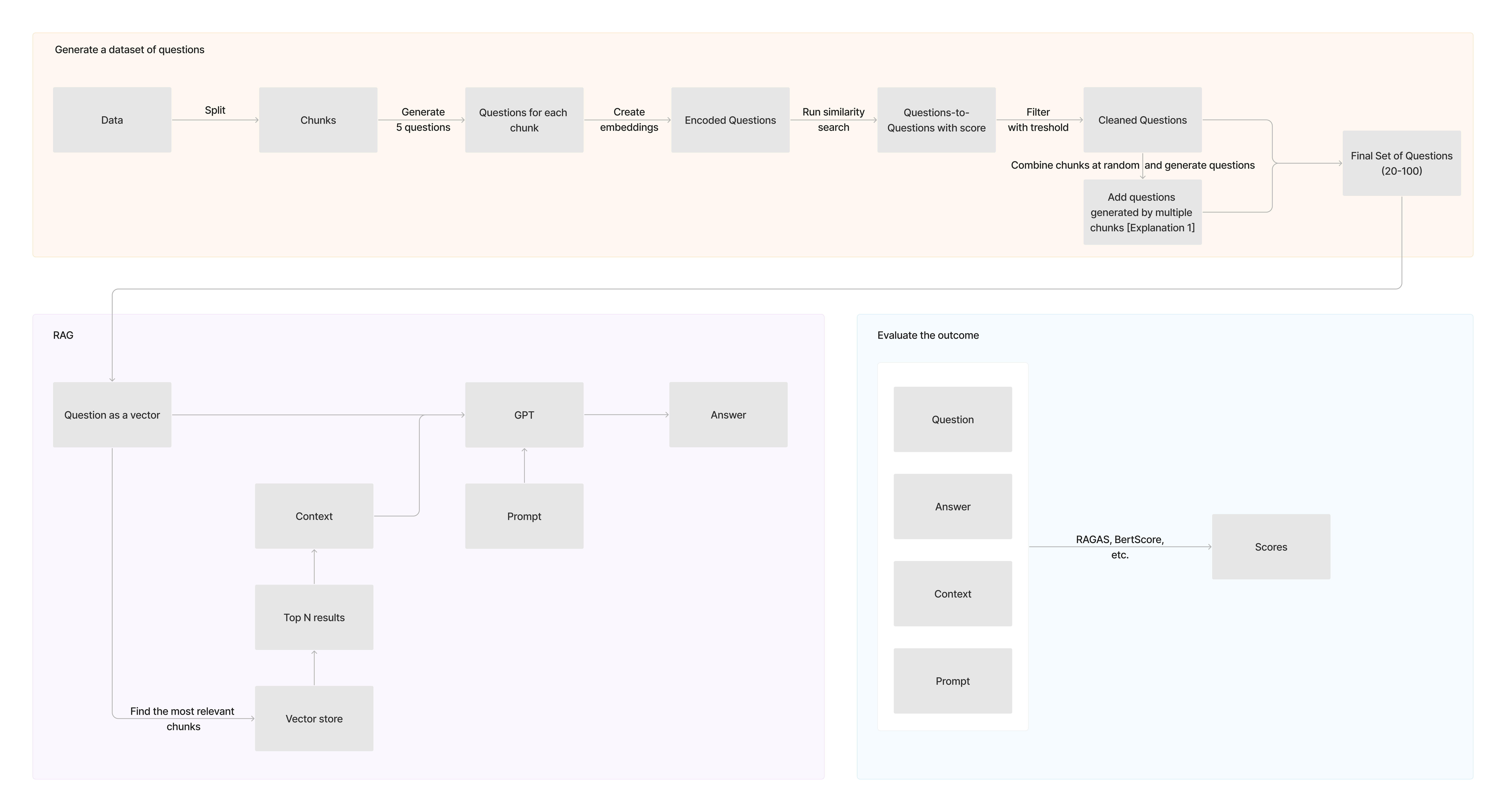
Evaluating RAG models involves a systematic process that can be broken down into several steps. Below is a detailed guide based on the key components.
Question Generation
- Generate N questions: with a simple prompt generate a set of N questions from each data chunk.
- Embed Questions: remove duplicated questions and convert the generated questions into vectors to perform similarity search later.
- Questions-to-Questions with Score: do question to question similarity in order to understand which questions are similar to each other. We would like to have only unique questions in the final set.
Question Filtering and Finalization
- Filter with Threshold: set a threshold to filter out questions that are very similar to each other to ensure question uniqueness and relevance.
- Combine chunks at random and generate questions:
- Integrate questions from various chunks randomly to enhance diversity and create a final set of questions.
- While developing questions from individual chunks of text, we encountered an issue where each question is linked to a single, isolated chunk. In reallity, it is frequent the case that question is answered by chunks that are connected between each other. To enhance our question set, we’re now focusing on formulating questions that draw upon multiple chunks, even if these chunks are located far apart.
- From Questions-to-Questions with Score step we know which chunks generates similar questions, so we could assume that these chunks could be connected to each other and be helpful while answering question. Finnaly, we could create questions that are based on multiple relevant chunks, combining relevant chunks and then use a language model to generate questions based on these chunks.
- Add questions generated by multiple chunks: Incorporate questions that are common across multiple chunks for robustness.
- Final Set of Questions (20-50): I would suggest to have a small set of questions in the beginning, to be able to evaluate quality visually yourself. Then you could increase the number of questions.
RAG
- Find the Most Relevant Chunks: Search in the vector store the most relevant chunks related to the question.
- Answer: Utilize GPT to generate an answer based on the question, given prompt and the context provided.
Evaluate the Outcome
- Score = Metric(Question, Answer, Context, Prompt)
- There are many metrics which could be applied to measure the quality of the result. I listed some that I found useful, you can find a list of metrics below.
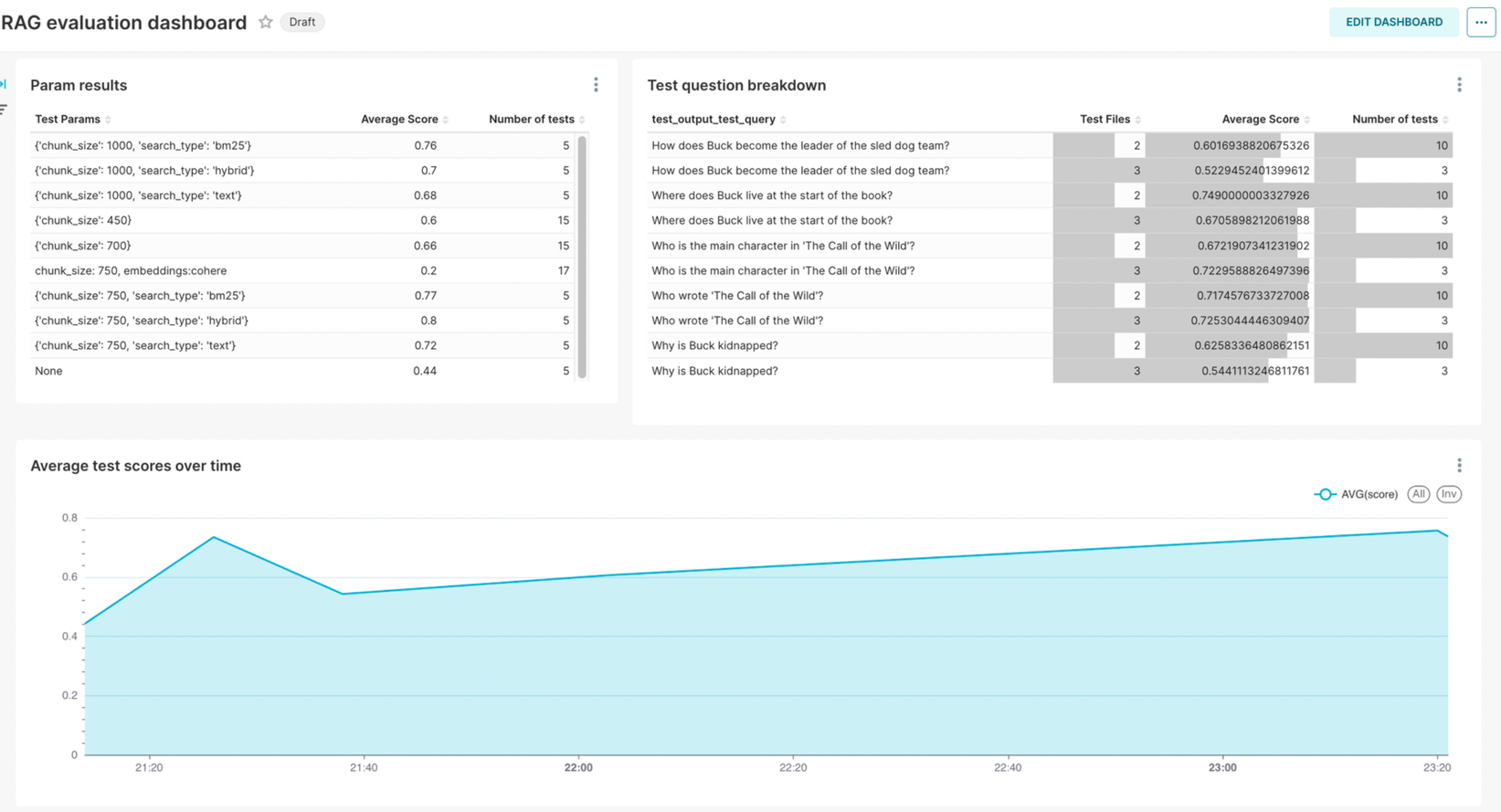
Parameters to optimize
There are a bunch of parameters that could be optimized to improve the quality of the final result. I listed some of them below.
Chunking | Retrival | Generation |
---|---|---|
Chunk size | Number of results | LLM Model |
Chunk overlap | Similarity threshold | Prompt |
Embedding model | Retrival Strategy (BM25, cosine similarity, hybrid) | Temperature |
Reranking |
Metrics
While there are numerous useful metrics, it’s impractical to focus on all of them simultaneously. The table below presents these metrics, which can be further categorized based on evaluation speed: slow
(taking approximately 5-10 seconds due to an additional call to the LLM for calculation) and fast
. This categorization aids in understanding the time efficiency of each metric during evaluation.
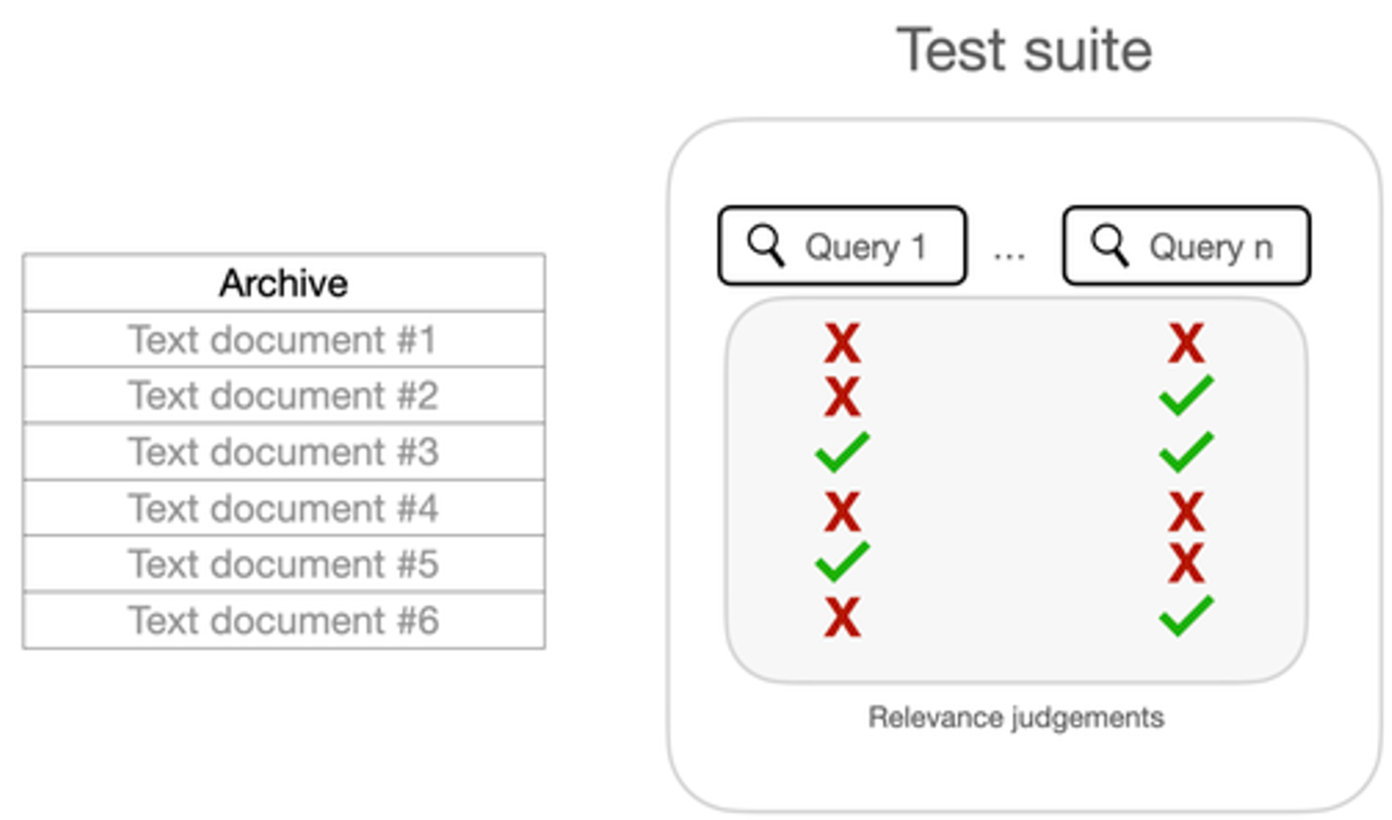
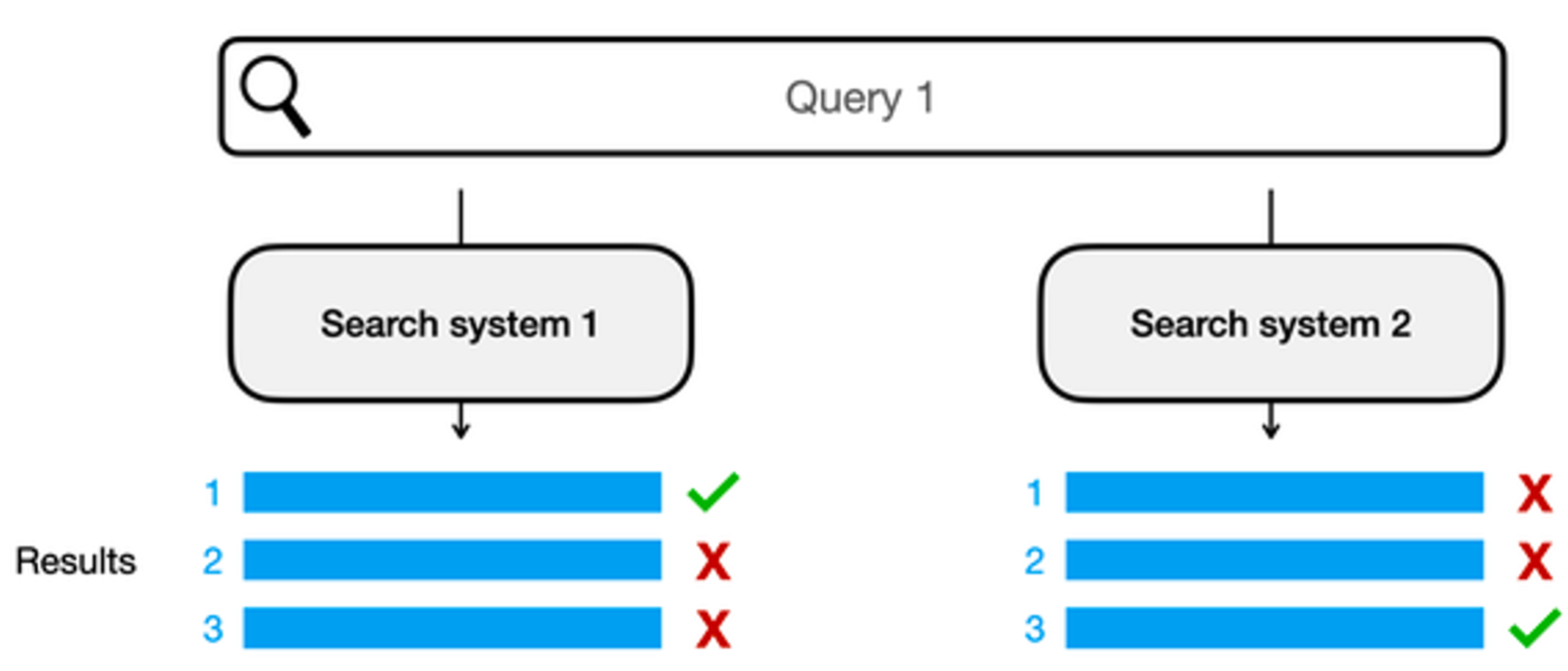
Chunking | Retrival | Generation |
---|---|---|
this is difficult to measure, therefore we can only evaluate end-to-end performance. | Context Precision (slow): Evaluates whether all of the ground-truth relevant items present in the context are ranked higher or not. Ideally all the relevant chunks must appear at the top ranks. This metric is computed using the question and the contexts . |
BLEU/ROUGE/BERTScore |
Mean Average Precision | Faithfulness (slow): This measures the factual consistency of the generated answer against the given context. It is calculated from answer and retrieved context . |
|
Normalized Discounted Cumulative Gain (nDCG) | Answer Relevance (slow): focuses on assessing how pertinent the generated answer is to the given prompt. This metric is computed using the question and the answer . |
Problems
- Problem: Evaluation Speed of Metrics
Current evaluation process is slow, taking approximately one minute per question for all metrics.
Solutions:- Parallel Processing: Run evaluations in parallel for each question, you can have multiple tokens for openai to achieve this.
- Threading: Utilize threads in newer models to enhance processing speed.
- Problem: Cost-Effectiveness and Efficiency
Due to the time-intensive nature of the analysis and associated costs, it is crucial to identify and address issues early in the process.
Solutions:- Fast Fail Approach: Implement mechanisms to quickly determine if there are problems with the system. You do not need to run costly tests if the system is not performing well on a basic level.
- Two-Tiered Evaluation Process:
- Fast Test Set: Begin with a quick evaluation using a top 20 question set that your system should be able to handle. If the set meets a threshold, i.e. 90% accuracy on specified metrics, proceed to a more comprehensive analysis.
- Extended Evaluation: Reserve longer, more detailed tests for periods following major releases rather than after every commit or pull request. This approach ensures that the end product is polished for customer use.
- Problem: User Interaction Analysis
Solutions:- Analytics Integration: Implement analytics tools, like Langfuse, to monitor and understand how users interact with your RAG.
- Problem: Relevance of Questions
Solutions:- Question Clustering: Organize questions into cluster groups and prioritize evaluation on the top 5 clusters.
- Topic Modeling: Employ techniques like BERTtopic to effectively group questions into relevant topics for targeted evaluation.
Quick wins to boost quality
- Embeddings are important to boost quality. Right off the box, you can get a 5-7% increase. You can tune the encoder end-to-end without tuning the LLM, you need to keep an eye on end metric for quality. You can look into MTEB (Massive Text Embedding Benchmark) to compare quality. Tuning with LLM should be the last resort.
- MMR(Maximal Marginal Relevance) on top of the Embeddings output increases the diversity of the final hints and has a better impact on the final generation. The idea here is that we need to get not the top N closest chunks, but the top N diverse relevant chuncks that are not paraphrases of each other.
- Hierarchical chunks splitting: from small to large. Specifically, we split the documents not into large pieces but maintain a hierarchy of large chunk - subchunks. Then we search specifically by subchunk, and in the prompt, the parent large chunk is returned as feedback. This expansion of context solves the ‘lost in the middle’ issues.